Most likely, you've heard this quote:
Data is the new oil.
This is actually part of a longer idea, usually credited to British mathematician Clive Humby:
Data is the new oil. Like oil, data is valuable, but if unrefined it cannot really be used. It has to be changed into gas, plastic, chemicals, etc. to create a valuable entity that drives profitable activity. so, must data be broken down, analysed for it to have value.
This analogy took off when The Economist published an article in 2017 titled "The world’s most valuable resource is no longer oil, but data". It pointed out that the biggest companies at the time weren't oil giants—they were data companies. The message was clear: if oil powered the industrial era, data is powering the digital one. That’s where the now-ubiquitous phrase “data is the new oil” came from.
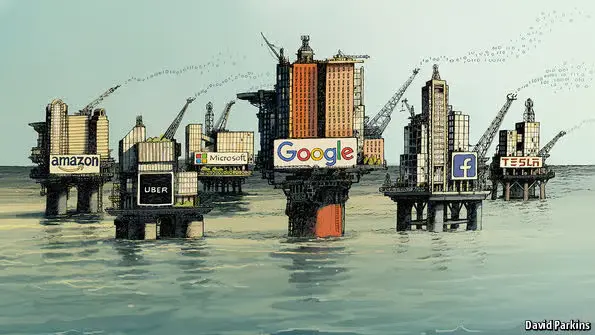
You could, of course, argue that data and oil aren't the same thing. Data isn't scarce. It can be copied, reused, and shared without losing quality. You can't just steal oil out of a pipeline, but, but data breaches happen almost every day.
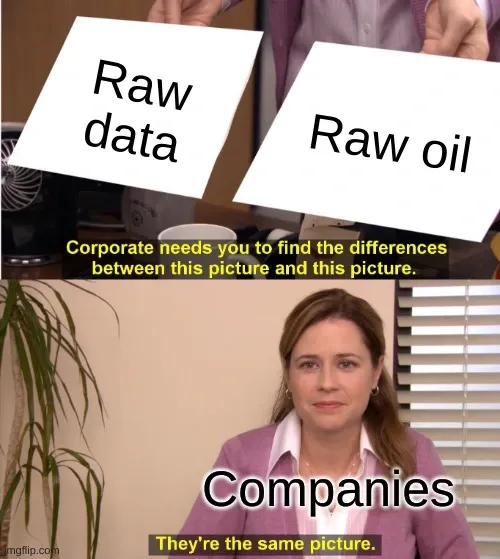
But for this post, that debate isn't the focus. What matters is this: data is now at the heart of the economy—and just like oil, it only becomes valuable when it’s processed.
Raw vs. Refined: Not All Data is Useful
Not all data is created equal. Just like crude oil, raw data is messy and not useful on its own. It's being generated constantly—by your phone, your browser, your smart watch, your camera. Every swipe, every click, every location ping is data. But most of it is unstructured, unlabeled, and—by itself—meaningless. Without any organization, context, or labeling, it’s just noise.
What makes data valuable is what you do with it. You have to sort it, clean it, tag it, and make it understandable to machines. You have to refine it. Just like oil must be turned into gasoline or plastic to become useful, data needs annotation, structuring, and validation before it can feed into AI systems or even basic analytics. That process—turning raw input into something structured and usable—is where the value comes from.
That’s how most of today’s tech companies make money. That’s how they build better products, smarter services, and more personalized experiences. Especially with AI and machine learning, refined data is what gives them a competitive edge.
Why Processed Data Powers AI
Everyone is talking about AI. Governments want to regulate it. Companies want to use it. Startups want to build on it. The cultural, economic, and political waves it’s creating are hard to ignore. It might actually be a legitimate turning point in history.
But here's the part that’s often glossed over: AI runs on data. Lots of it. These models—however advanced or mysterious they seem—are just very powerful statistical systems that learn from examples. For instance, GPT-3 was trained on roughly 45 terabytes of compressed text. After filtering, that came down to about 570 gigabytes of training data. That's still massive.
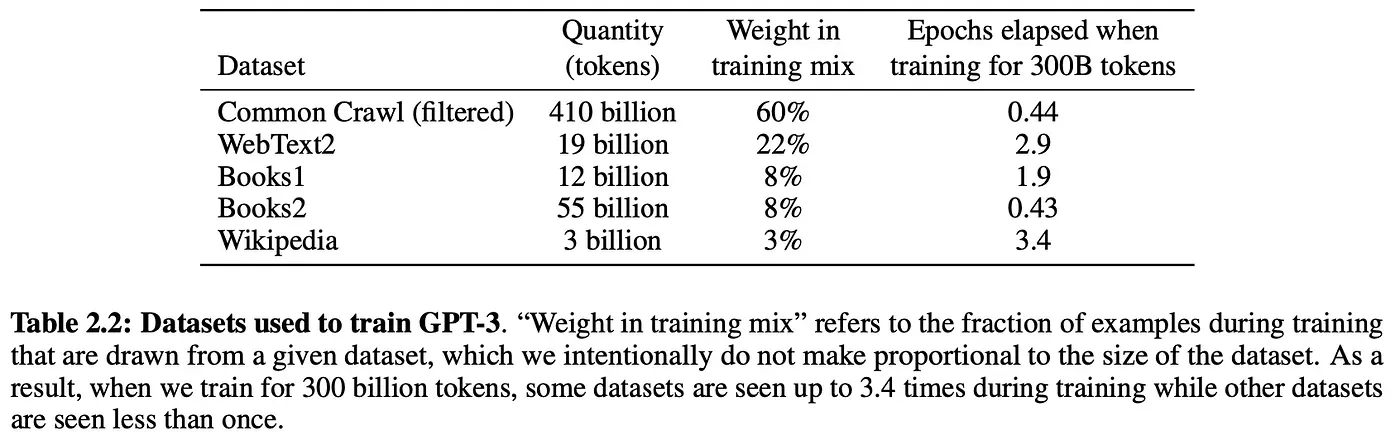
There's also a general rule of thumb: the more complex the model, the more data it needs. In fact, the usual estimate is a 10:1 ratio between the number of parameters a model has and the number of training examples it needs. That’s a huge amount of data.
But again, it's not just about size—it's about quality. Models don't learn well from random, raw data. They learn from labeled examples. Whether you're training a model to identify lung cancer in X-rays, detect objects in self-driving cars, or translate speech into text, you need annotated, accurate, human-reviewed data.
That’s why a lot of AI breakthroughs don’t come from better algorithms—they come from better datasets. If AI is the engine, refined data is the fuel. And the cleaner, more specific, and more accurate the data is, the better that engine runs.
Challenges in Processing Data
Let's stick with the oil metaphor a bit longer: refining oil takes work. You need infrastructure, money, people, and time.
Same goes for data. Processing it—cleaning it, storing it, securing it, annotating it—isn't free. It's hard. Data comes in all shapes and formats: structured, unstructured, semi-structured. It’s scattered across systems and platforms. You have to bring it all together before it even makes sense.
One of the hardest parts? Data annotation. It's slow. It's expensive. And in many cases, it can't be fully automated. You need humans—often domain experts—to look at text, images, video, or audio and label it correctly. That labeling has to be consistent. It has to avoid bias. And it has to respect privacy and compliance regulations like GDPR.
No surprise then that the last few years have seen a boom in data labeling tools and startups. Scale AI, Roboflow, and SuperAnnotate are leading names. There are also newer players like Unitlab AI and Labellerr, plus a growing set of open-source tools for teams who want more control.
In short: refining data is no easy task. But it’s necessary.
Conclusion
The phrase still holds: data is the new oil. But here’s the key point—it only matters if it’s processed. Unstructured, unlabeled, raw data doesn’t power anything.
Today’s AI race isn’t just about who has the biggest model. It’s about who has the best data. Because when everyone has access to the same open-source algorithms and similar computing power, what really sets you apart is the quality of your AI/ML datasets.
So the question isn’t whether you have data. Everyone does. The real question is: Are you refining yours? Or just sitting on a giant pile of digital crude that nobody can use?
Explore More
Explore these resources for further information on data and its applications:
- Computer Vision in Healthcare: Applications, Benefits, and Challenges
- Practical Computer Vision: Parking Lot Monitoring
- What is Computer Vision Anyway?
References
- Dennis Layton (Jan 31, 2023). ChatGPT — Show me the Data Sources. Medium: https://medium.com/@dlaytonj2/chatgpt-show-me-the-data-sources-11e9433d57e8
- Michael Abramov. (Jul 11, 2023). Data Is the Most Critical Item of 2023. Keymakr Blog: https://keymakr.com/blog/data-is-the-most-critical-item-of-2023/