In one of our earlier posts, we provided a comprehensive overview of the different types of image annotation solutions. Over time, we have been writing about each of them in detail, which you can find on our blog.
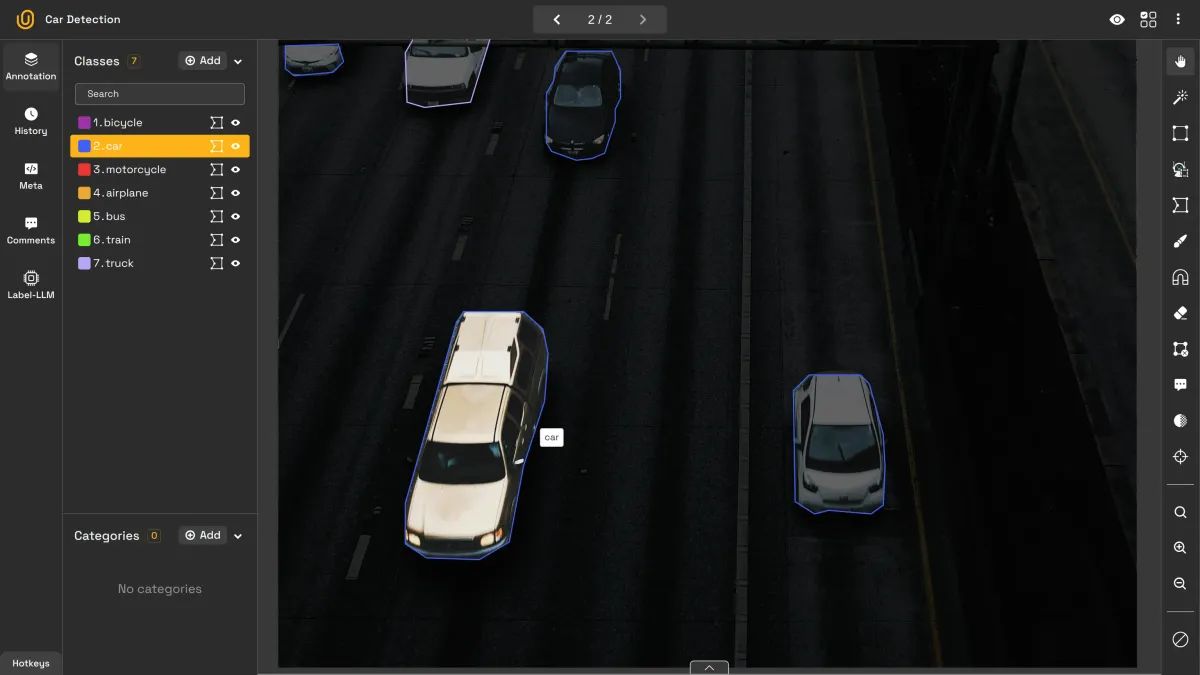
Image Annotation Types | Unitlab Annotate
Today, let’s focus on image point annotation, also known as keypoint annotation. It’s one of the specialized types of image labeling used by many data annotation service providers, known for its unique use cases and challenges.
Introduction
Think of point annotation as a crucial step in image labeling. By adding points to an image, you can specify landmarks that reveal shapes or positions. If you connect a few dots to create a joint structure, you essentially get a skeleton or blueprint of the object, referred to as image skeleton annotation. This skeleton comes essentially from image point annotation.
Both point and skeleton annotation differ markedly from traditional methods like bounding boxes and polygons. Image point and skeleton annotation specify unique landmarks inside the object, while bounding boxes and polygons outline it as a whole. Consequently, traditional methods simply show which parts of the image belong to which class, without detailing how those parts are arranged. By contrast, point and skeleton annotation clarify the object’s internal structure.
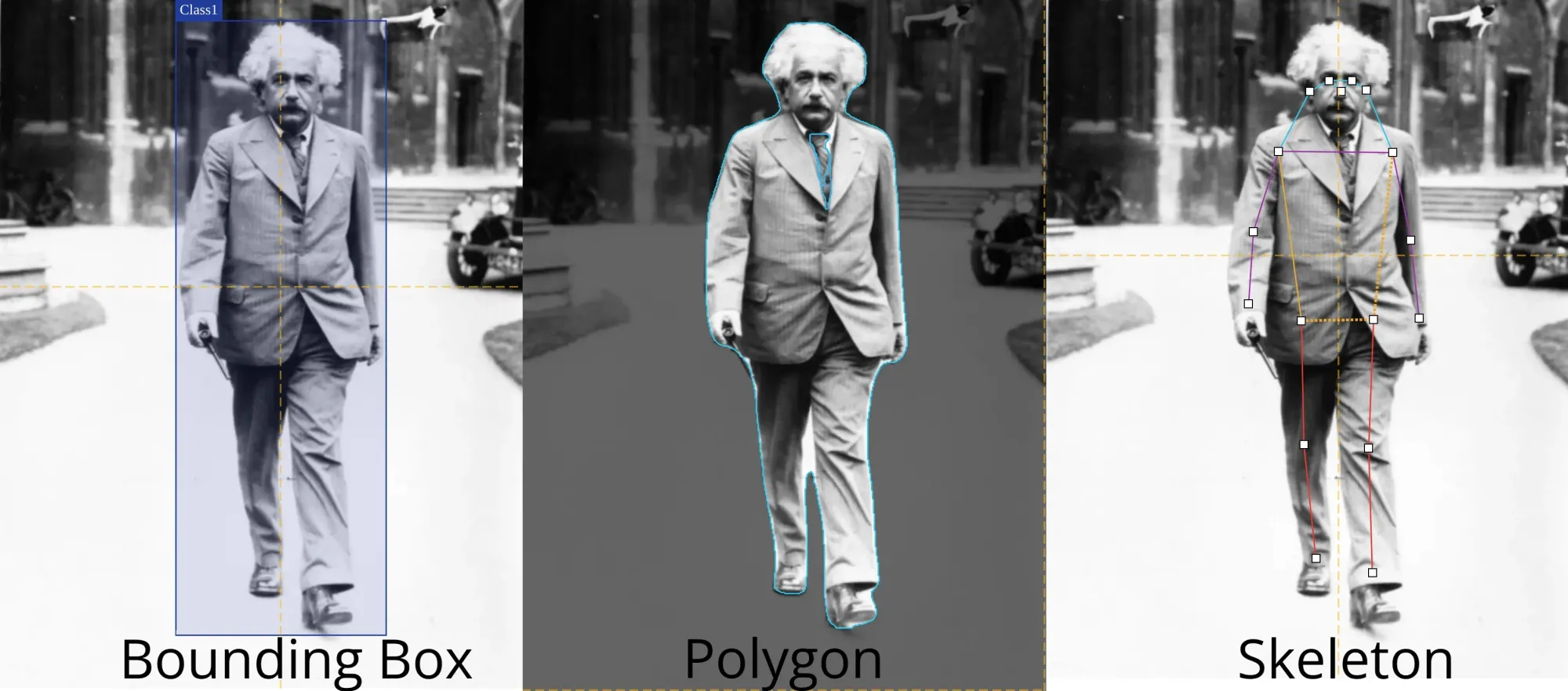
Each approach has its advantages. Point labeling is a relatively new but essential tool for pinpointing features and landmarks with high accuracy. The main focus here is spatial data—think of it like placing markers on your map to highlight points of interest, such as cities, especially when building datasets for geospatial analysis using AI.
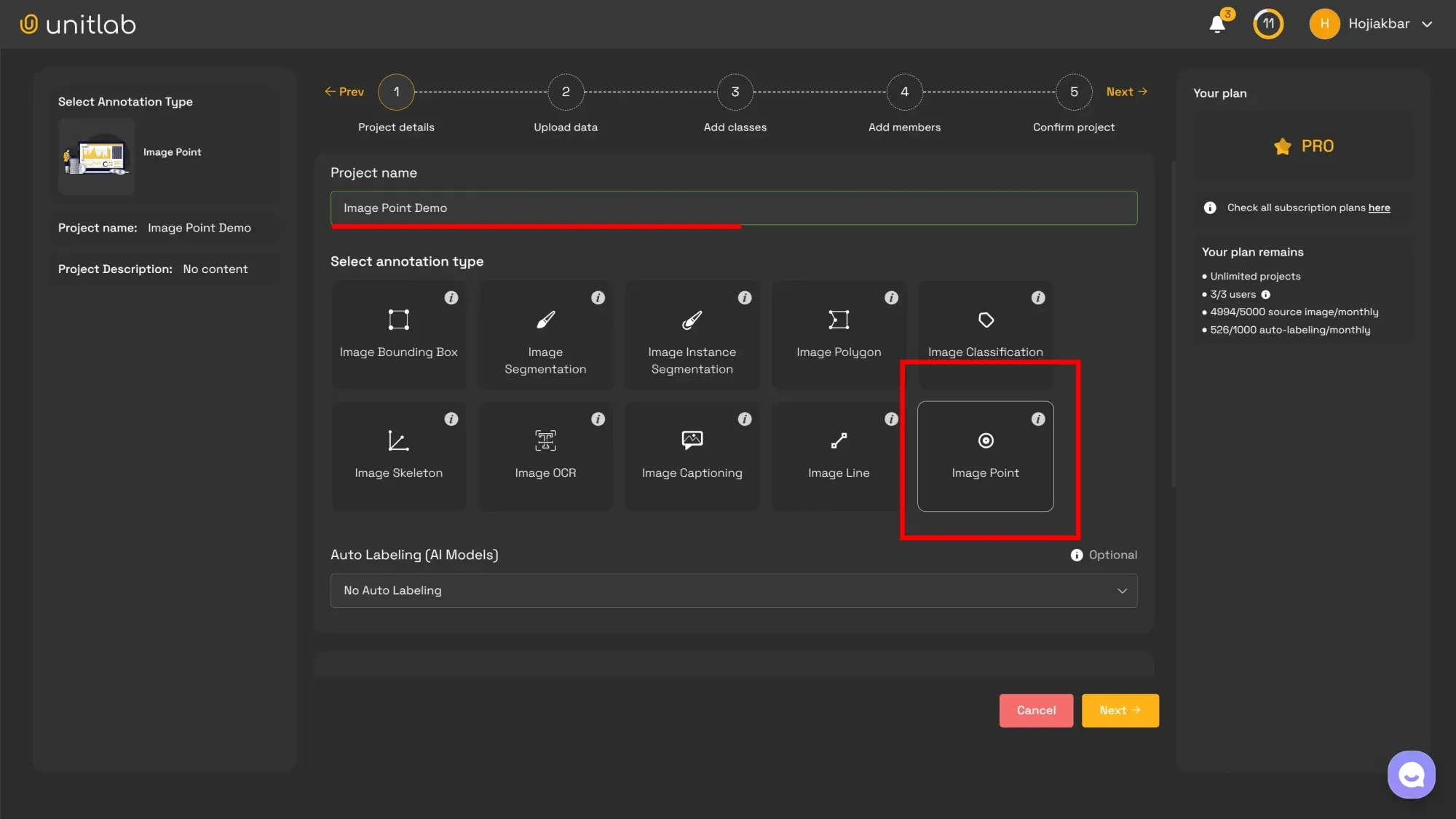
We are first going to create a project to visually highlight applications within Unitlab Annotate, an innovative data annotation platform.
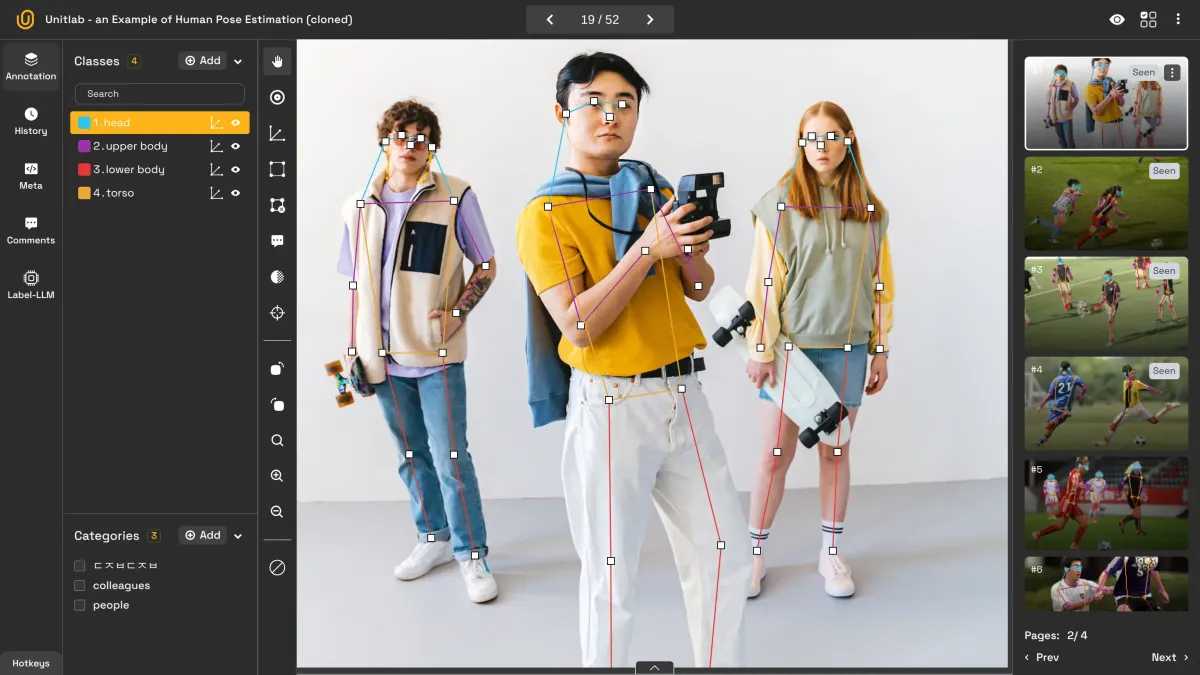
Project Creation | Unitlab Annotate
Image Point vs. Image Skeleton
We’ve previously written an in-depth guide on image skeleton annotation, which expands on image point annotation by connecting multiple points to represent objects. Although point and skeleton annotation are closely related, they serve slightly different purposes in computer vision.
Image Point Annotation
This technique focuses on marking specific, individual points in an image. You might highlight the tip of a pen, the corner of a box, or a knee joint. Each point exists independently, making it ideal for tasks requiring precise localization but no connection between points. Many data labeling tool providers incorporate this method for straightforward detection tasks. However, if you want a structured “blueprint” view of an object, you’d likely go with image skeleton annotation.
Face Landmark Point Annotation | Unitlab Annotate
Image Skeleton Annotation
In contrast, image skeleton annotation links these individual points to create a skeleton. For instance, in human pose estimation, you might connect keypoints (shoulders, elbows, wrists) to form a human body skeleton. This approach reveals the relationship between points—angles, movement patterns, and relative positions.
Using the map analogy: one marker might represent a small town or a single mountain, but multiple connected markers can outline a road or a mountain range. Your choice ultimately depends on the complexity of what you are annotating. In many data annotation solution workflows, skeleton annotation is especially popular for human pose tracking and movement analysis.
Key Differences
Summarizing the main contrasts between point and skeleton annotation:
- Focus: Point annotation highlights individual points, while skeleton annotation connects them in a “skeleton-like” format.
- Applications: Points excel at object localization, while skeletons are crucial for analyzing motion or poses.
- Complexity: Skeleton annotation typically needs more advanced algorithms and frameworks to ensure accuracy. Point annotation, by comparison, is simpler yet highly effective.
Recognizing these differences will help you select the right approach for your project.
Use Cases
Because image point annotation is both intuitive and capable of capturing crucial details, it has a range of real-world applications:
-
Facial Landmark Detection
- Identifying eyes, nose, lips for facial tracking or emotion recognition.
- Strengthening security via precise facial recognition.
-
Medical Imaging
- Pinpointing critical anatomical points to power diagnostic tools.
- Supporting research on diseases (e.g., arthritis) through joint analysis.
-
Autonomous Vehicles
- Detecting keypoints on road signs, pedestrians, and other vehicles.
- Contributing to safer, more efficient navigation systems.
-
Robotics and Manufacturing
- Teaching robots to locate keypoints on objects for better manipulation.
- Improving quality control by pinpointing defects in production processes.
In many image labeling workflows, these use cases are central for building robust machine learning pipelines across industries.
Challenges
While point annotation offers major benefits over conventional image labeling approaches in its applications, it also has its share of obstacles. Addressing these hurdles is vital for producing high-quality annotations and achieving optimal performance in computer vision models. Some challenges are point-specific, while some are general in image annotation:
-
Ensuring Precision and Accuracy
- Points must be placed with extreme accuracy, especially for fine-grained tasks like medical imaging.
- Inconsistent skills or ambiguous guidelines can create inconsistent results.
-
Occlusions and Ambiguity
- Overlapping or partially hidden objects complicate point placement.
- Clear instructions and well-trained annotators are crucial for complex scenes.
-
Scalability for Large Datasets
- Labeling thousands of images with numerous points is time-consuming without data auto-annotation or image auto labeling tools.
- Automated approaches can help but may not match manual accuracy in challenging scenarios.
-
Cost and Time Constraints
- High-quality annotation requires high investments in tools, training, and manpower.
- Balancing cost with the demand for precision remains a persistent challenge.
Overcoming these difficulties often involves advanced software, solid annotation standards, robust annotator training, and combining manual human labeling with auto labeling methods.
Conclusion
Point annotation (and, by extension, image skeleton annotation) is a precise and powerful technique in the image labeling solution arsenal. Its capacity to reveal intricate details—from basic localization to structural relationships—makes it indispensable in everything from healthcare to self-driving technologies.
By understanding this annotation method’s nuances and tackling its challenges head-on, teams can unlock its full potential. Like every annotation type, point annotation has its own specialized role and will remain essential for AI/ML models that need granular detail. When combined with robust dataset management, version control, or AI dataset management, these annotations can easily be used to prepare high-quality labeled image sets for training your AI/ML models.