What is pixel-perfect image labeling?
Pixel-perfect image labeling is a cutting-edge approach to image annotation that ensures every pixel in the interested object is accurately annotated. This method is capable of annotating every pixel in our object of interest as it captures literal pixels in the image. In comparison, polygon and bounding box tools first capture the coordinates of the outer edges of the object of interest and then "fill in" the area within those edges. Because of the difference in approaching image annotation (pixels vs. coordinates), their outputs and annotation process differ significantly.
Unlike traditional, common methods like bounding boxes or polygons, pixel-perfect data annotation captures even the finest details with outstanding accuracy. This precision makes pixel-perfect image annotation a critical tool for industries that rely on high-quality, precise AI and ML datasets, especially in the medical image diagnosis.
Traditional vs. Pixel-Perfect
The following shows the image annotation with a traditional polygon tool. The object of interest (sweater) is outlined using the polygon tool, but the tool does not fully cover the sweater. The annotation may cover areas that do not belong to the object; conversely, it may not fully cover the object, hence the imperfect-pixel image annotation. Although quite common, this tool cannot produce high-quality datasets that have a huge number of irregular or round objects. Here is how a human pose would traditionally be annotated with the polygon tool in a data annotation platform:
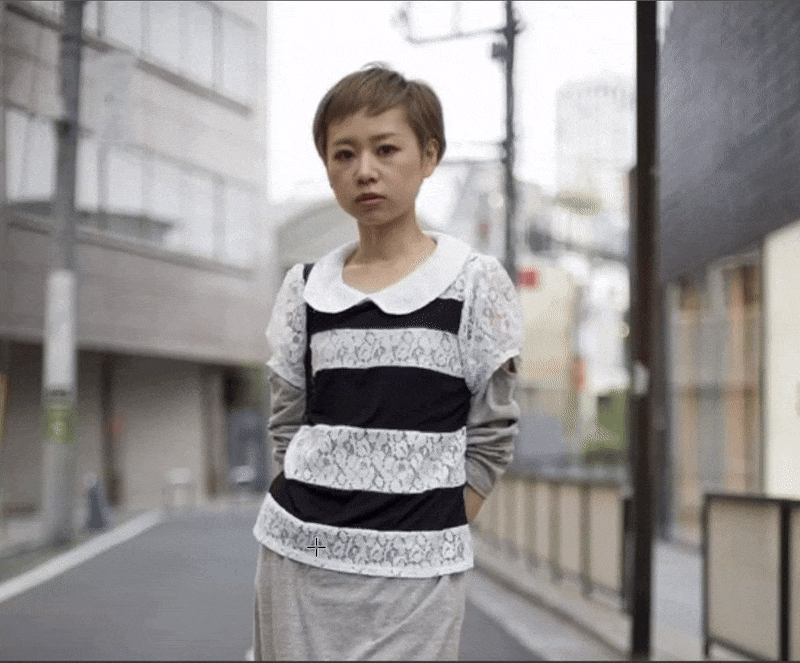
In this example, however, every pixel in our object (sweater) is fully annotated, resulting in 'pixel-perfect image annotation'. Notice how a brush segmentation tool is used to annotate every pixel in the sweater in Unitlab Annotate. It can be used both for regular objects, such as rectangles, and irregular objects, such as human poses, round objects, and others. See how easy and precise it is with the brush tool to perfectly annotate any object:
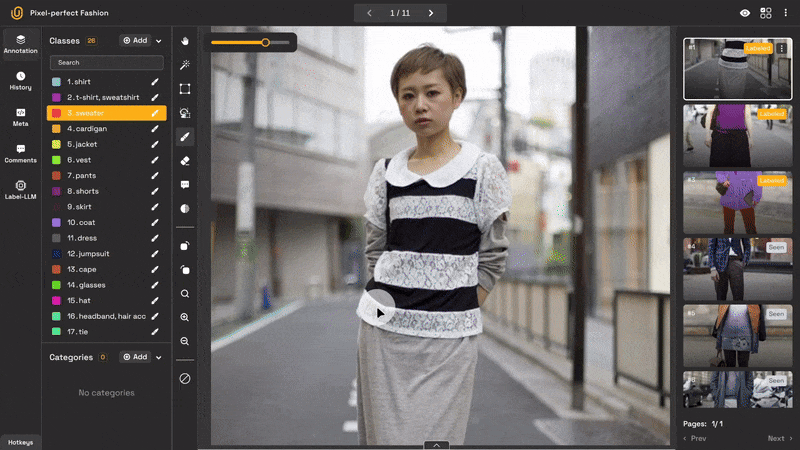
Almost every data annotation platform offers traditional methods of image labeling. While, in theory, it is possible to achieve pixel-perfect segmentation with traditional polygons and bounding boxes, it takes too much effort and time for the human data annotator. The precision is also lower than that of advanced techniques. This video shows how a polygon tool in a data annotation platform would be used to annotate a complex, irregular shape:
Imperfect Pixel Segmentation in Current Tools
With pixel-perfect image labeling tools, the time spent labeling images reduces significantly, while the precision of image annotations increase, due to ease of use and inherent precision in pixel-perfect annotation tools. The following video shows how the same complex, irregular human pose can be annotated faster and easier with pixel-perfect annotation tool with Unitlab Annotate's brush instance tool:
Brush Instance Segmentation | Unitlab Annotate
What's more, AI-powered auto-annotation tools, namely the SAM (Segment Anything Model), can be used for pixel-perfect annotation as well. While brush and magnet tools offered by Unitlab Annotate let you create pixel-perfect image annotations, AI-powered auto-annotation tools enable you to significantly reduce the time spent annotating and cut the costs at the same time.
In other words, the step-by-step, efficient transition from traditional image annotation to pixel-perfect image labeling would be:
- Traditional tools (the polygon)
- Pixel-perfect tools (the brush)
- AI-powered pixel-perfect annotation (the SAM).
The third way is very simple to use. A human image annotator just clicks the area of interest and the model itself makes the annotation. Let's see how it would work for our complex, irregular human pose:
Pixel-perfect Annotation with the SAM | Unitlab Annotate
Annotating Round Objects
Another issue with traditional annotation methods arise when we annotate rings and circles. It is virtually impossible to annotate rings and tires accurately with polygon or bounding box annotation. This type of image annotation covers the whole object, making it difficult for us to specify space inside the object of interest.
For example, in the first image, we have to use points to mark the edges of a circle - a difficult task to achieve with high accuracy. Even if we drew the perfectly precise annotation using the polygon tool, our tool would annotate the whole tire, which is inaccurate and confusing for our future AI/ML models. By comparison, we can easily draw annotations around the tire using the brush tool with outstanding precision and speed. Our annotations only include the pixels that are actually drawn using the brush segmentation tool.
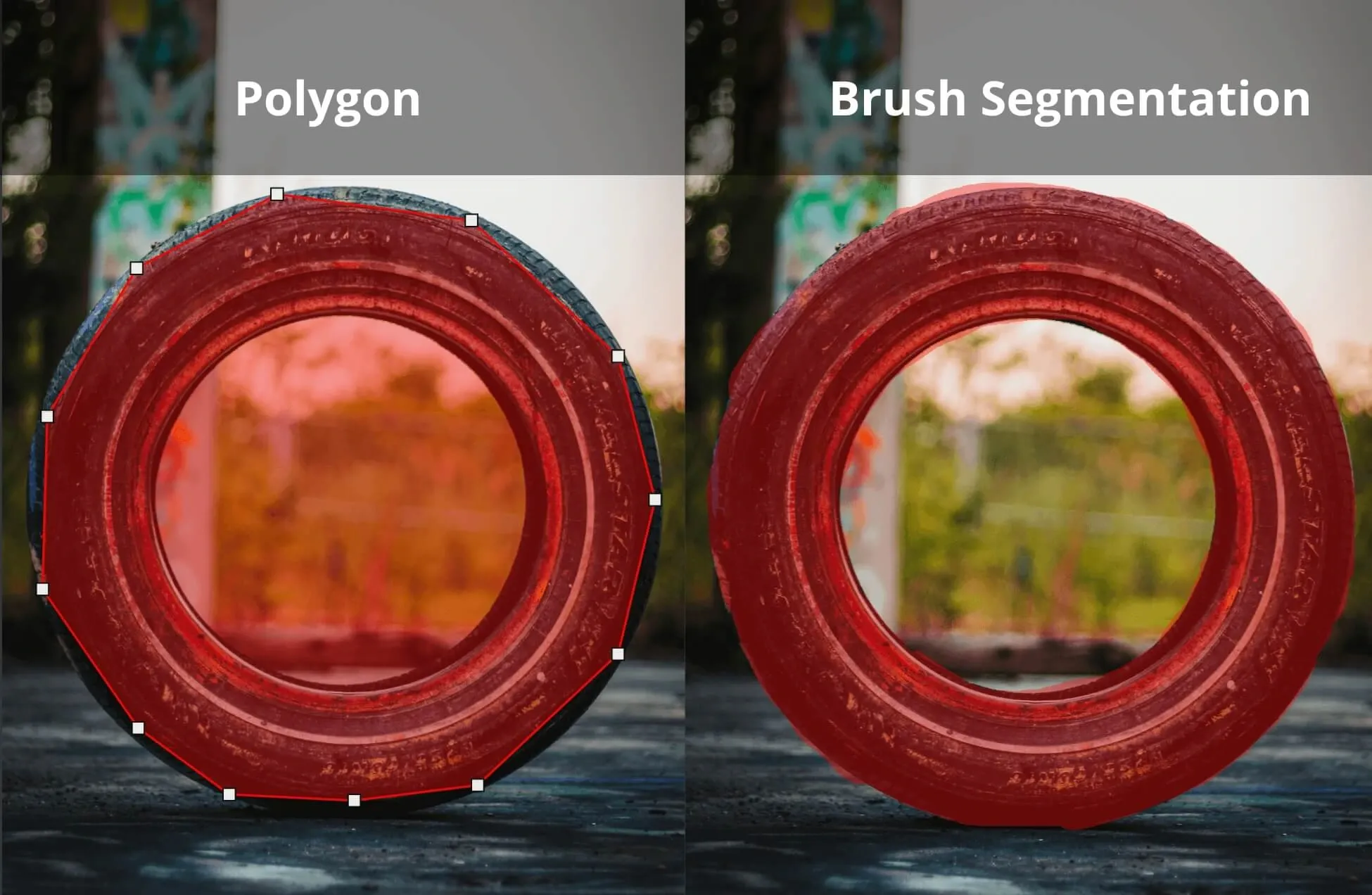
Benefits
So, in short, pixel-perfect image annotation solutions are beneficial for several reasons:
-
Unmatched Precision: By labeling objects pixel by pixel, this approach ensures the highest level of accuracy, which is crucial for complex tasks like tumor segmentation or lane detection. This level of detail is what sets pixel-perfect data labeling solutions apart from more basic/traditional methods.
-
Enhanced Model Performance: Machine learning models trained on pixel-perfect image data annotation consistently perform better, particularly for tasks that require detailed object boundaries. When paired with proper dataset management and dataset version control, the results are even more robust.
-
Clear Object Boundaries: Pixel-perfect data labeling eliminates ambiguity, making it easier to differentiate between overlapping or closely packed objects. This is essential for building reliable AI datasets and optimizing ML datasets.
Solutions
You are most likely to use a data annotation platform to streamline your image annotation process. It is essential to compare these platforms in terms of features and pricing, especially when not many platforms offer pixel-perfect segmentation.
To help you explore key features and pricing plans of various data annotation platforms, earlier this year, we made a cross-comparison between 12 top leading data annotation solutions. We believe that it is essential to choose the ideal data annotation platform for your use cases.
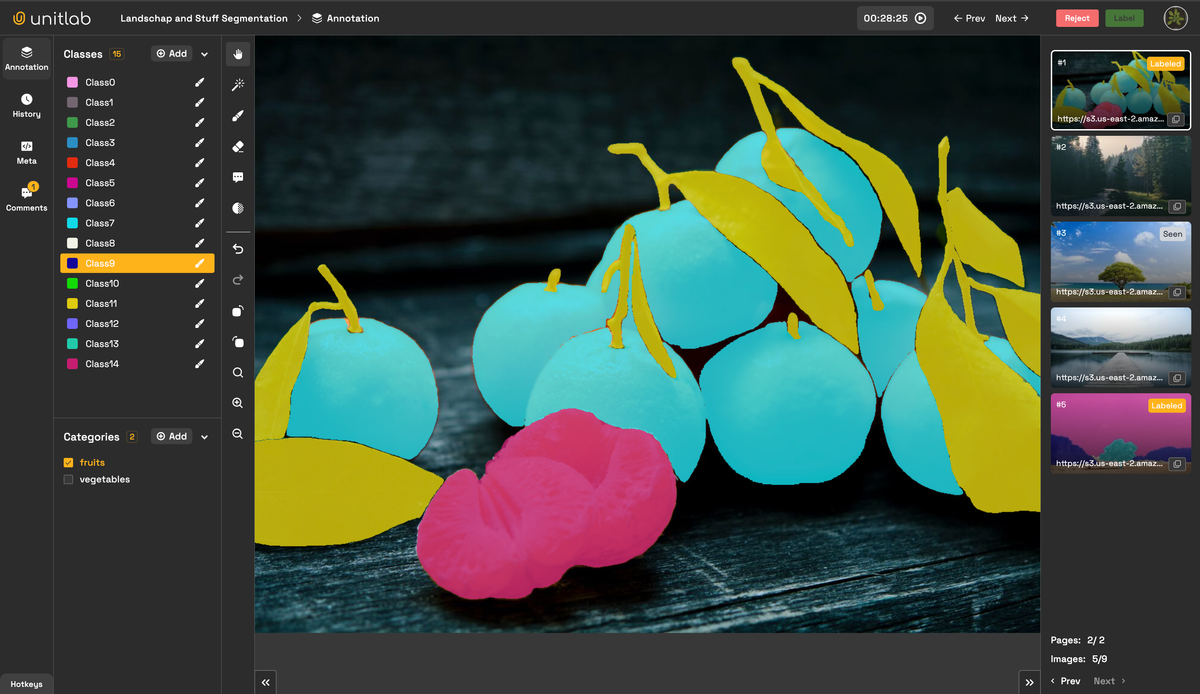
Top Image Annotation Tools of 2024 | Unitlab Annotate
As the pixel-perfect segmentation is a new and evolving method of image annotation, we can see that there are not many data annotation platforms that offer the pixel-perfect image labeling, as shown in our infographic:
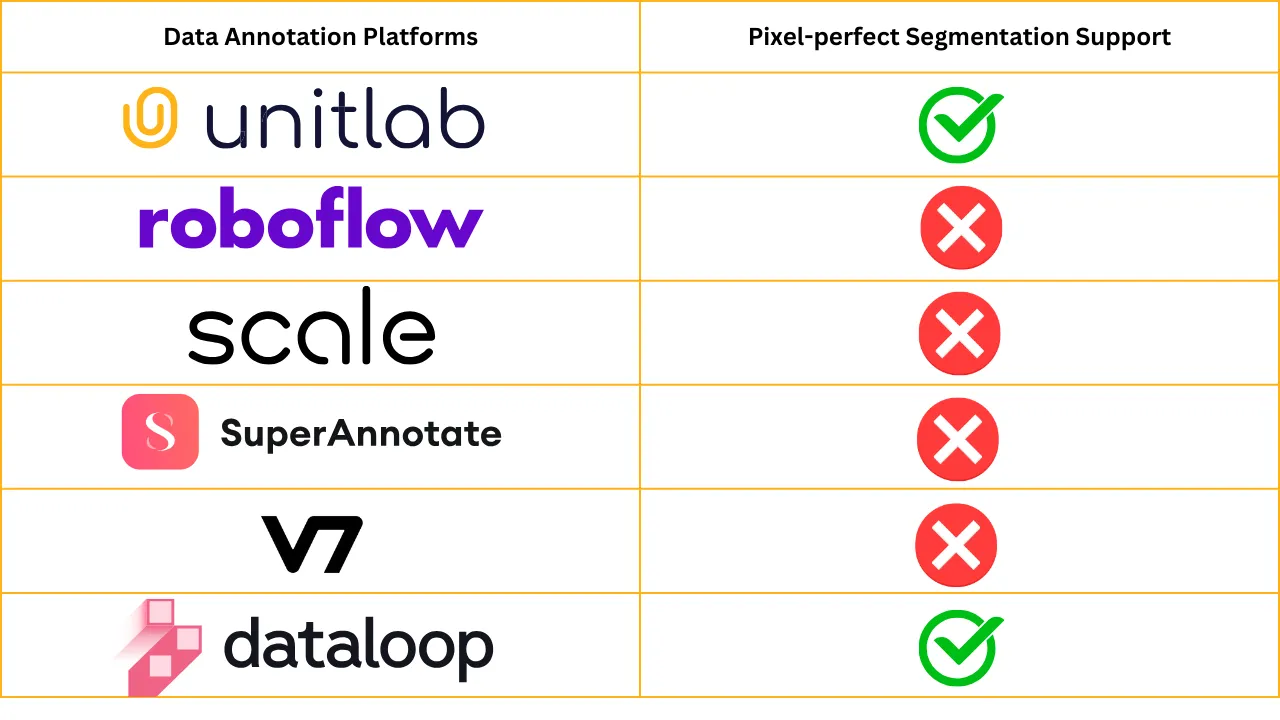
One of the distinct features of Unitlab Annotate, among others, is that it provides pixel-perfect image annotation tools, both manual and AI-powered automatic, to be used in your image annotation workflows and computer vision projects. It offers two types of pixel-perfect segmentation tools:
- Image Segmentation
- Image Instance Segmentation
Tools
To achieve pixel-perfect precision, Unitlab Annotate image labeling tools offer various methods and features, both manual and AI-powered automatic. First, let's create a project and choose the right type of image annotation.
While creating a project, make sure to choose one of these image annotation types. You can choose to use built-in AI models to help you automate pixel-perfect image annotations. We will first explore how to draw manual pixel-perfect image annotations.
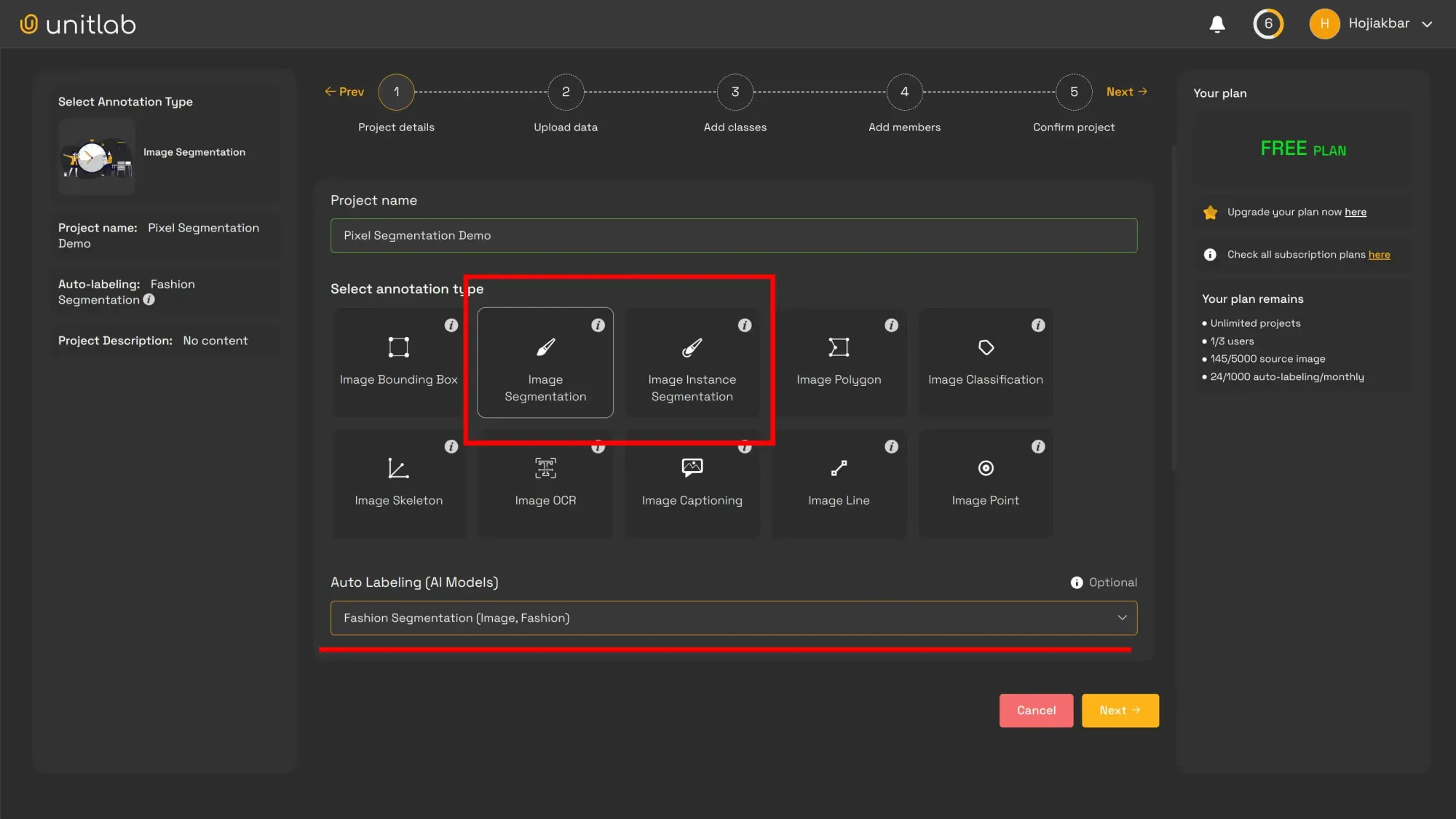
Brush Segmentation
Brush segmentation, as part of the image segmentation, is one of the simplest yet most effective techniques for data annotation. Human annotators use a brush-like tool to mark every pixel of the object. They essentialy brush the area/object that should be annotated.
It is also possible to erase any parts of the annotated object if the annotation is outside your object. You can annotate irregular objects, which traditional methods of image annotation cannot, with high accuracy and speed:
Brush Segmentation | Unitlab Annotate
- Ideal For: Irregularly shaped objects requiring detailed labeling.
- Features: Adjustable brush size and zoom controls for pixel-level precision.
- Key Benefit: A flexible, yet straightforward image labeling solution for achieving exact results.
Brush and Magnet Tool
The brush tool and the magnet tool from the brush instance segmentation are another option that Unitlab AI provides for pixel-perfect image annotation. While the eraser tool lets you erase incorrect annotations, the magnet tool lets you add and erase annotations from your current brush instance annotation.
For example, you may annotate 80% of the image, but if you annotate the remaining 20% with a new annotation, the model sees them as two different annotations even though they may seem alike to the human eye. The magnet tool helps the human data annotator to add and remove annotations. This ensures that image labelers can annotate the object precisely and clearly, and AI/ML models can use high-quality datasets.
Brush and Magnet Tool | Unitlab Annotate
Magic Touch: SAM auto-annotation
So far, we have seen only manual pixel-perfect annotations. Unitlab Annotate offers many AI-powered auto-annotation tools. the Magic Touch, a pixel-perfect auto-annotation tool powered by the Segment Anything (SAM) model, uses a polygon tool under the hood. However, this SAM can still annotate any object with outstanding precision, regardless of its structural complexity, ensuring pixel-perfect accuracy. The image labeler just clicks the area of interest, and the model automatically detects and annotates it. Let's see the demo with the dental image instance segmentation:
Magic Touch Annotation | Unitlab Annotate
Auto Labeling and Data Annotation Automation
For larger projects, data auto-labeling and auto-labeling tools of pixel-perfect segmentation can significantly speed up the process. Unitlab Annotate offers batch and crop auto-annotation to automate image labeling. These two features utilize the current AI-model in your project, which can be the pre-built models provided by Unitlab Annotate or your custom models integrated into Unitlab.
These tools combine AI-driven automation with manual refinement, offering a scalable and efficient data annotation solution. By integrating image auto-labeling tools, your team can handle more complex datasets while maintaining quality.
Conclusion
Traditional methods of image annotation are widespread, familiar, and easy to understand, but it is difficult to achieve high precision and speed with them. They are not the right types for some of the more complex images and shapes.
Pixel-perfect image annotation solutions represent the highest standard in image annotation services. With tools offered by Unitlab Annotate - brush segmentation, brush instance segmentation, and SAM auto-labeling tools, it is easy and fast to achieve precise results. No matter how complicated and irregular your objects may be, Unitlab Annotate can streamline the difficult process of annotating these objects.
Whether you’re looking for a comprehensive data labeling service or exploring advanced image labeling tools, Unitlab Annotate ensures that your data meets the highest standards with its comprehensive pixel-perfect segmentation tools and AI models.