Agriculture is one of the oldest and most vital human endeavors. But today, agriculture faces mounting pressure from a growing global population, shifting climate patterns, and the need to adopt more sustainable practices. These challenges are pushing the agricultural sector toward smarter, more efficient solutions—and technologies like artificial intelligence (AI), the Internet of Things (IoT), and computer vision are leading the way.
One of the most promising technologies making an impact is computer vision, a field of AI that enables machines to interpret and analyze visual information from images and videos—just as humans do.
Once reserved for high-tech industries like healthcare or security, computer vision is now playing an increasingly important role in agriculture. With the support of AI, IoT devices, and necessary systems and the infrastructure, this technology is helping farmers boost productivity, cut costs, and tackle global challenges like food security and sustainable resource management.
In this article, we’ll take a closer look at:
- What computer vision is
- How it’s being applied in agriculture
- What the benefits and challenges are
What is Computer Vision?
At its core, computer vision is about teaching machines to “see.” More specifically, it’s a branch of AI focused on enabling systems to extract meaningful insights from visual data—like images or video feeds—and act on them. When paired with machine learning algorithms and well-curated datasets, these systems can recognize patterns, make predictions, and drive real-time decision-making.
In agricultural applications, computer vision models are leveraged through robust datasets, ML algorithms and hardware that perform:
- Object detection: Spotting weeds, pests, or different crop types
- Image classification: Distinguishing between healthy and diseased plants
- Segmentation: Mapping distinct regions in a field for targeted intervention
These tasks require high-quality datasets to ensure models continue to perform under varied real-world conditions.
Applications of Computer Vision in Agriculture
The quest for CV and AI usage in agriculture stems from a variety of factors. The global population increase and declining arable land mean that agricultural productivity (output per hectare land) must increase in order to feed the human population in the coming decades.
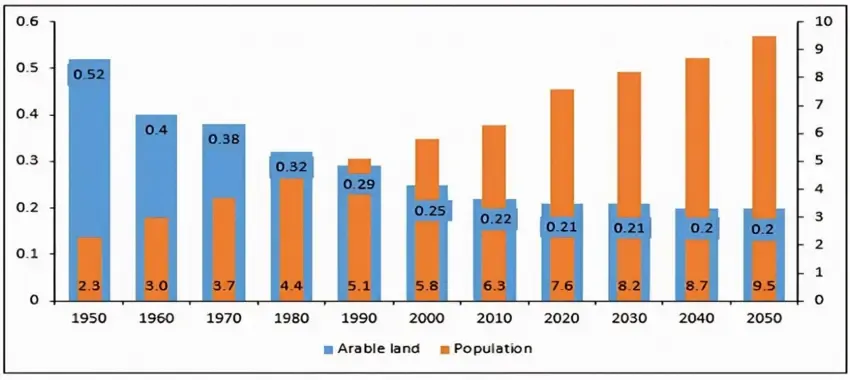
Modern problems require modern solutions. There have been proposed many innovative ideas and subsequent startups regarding the global agricultural issues, from vertical farming to drip irrigation to vision-based intelligent systems.
The focus of the present day research in agriculture is the development of intelligent and automated vision-guided systems that can replace manual processes and are more precise and accurate. A vision-guided intelligent system must be able to gather relevant information without human intervention, consequently reducing labor cost. We are going to explore applications and techniques of computer vision and AI in agriculture.
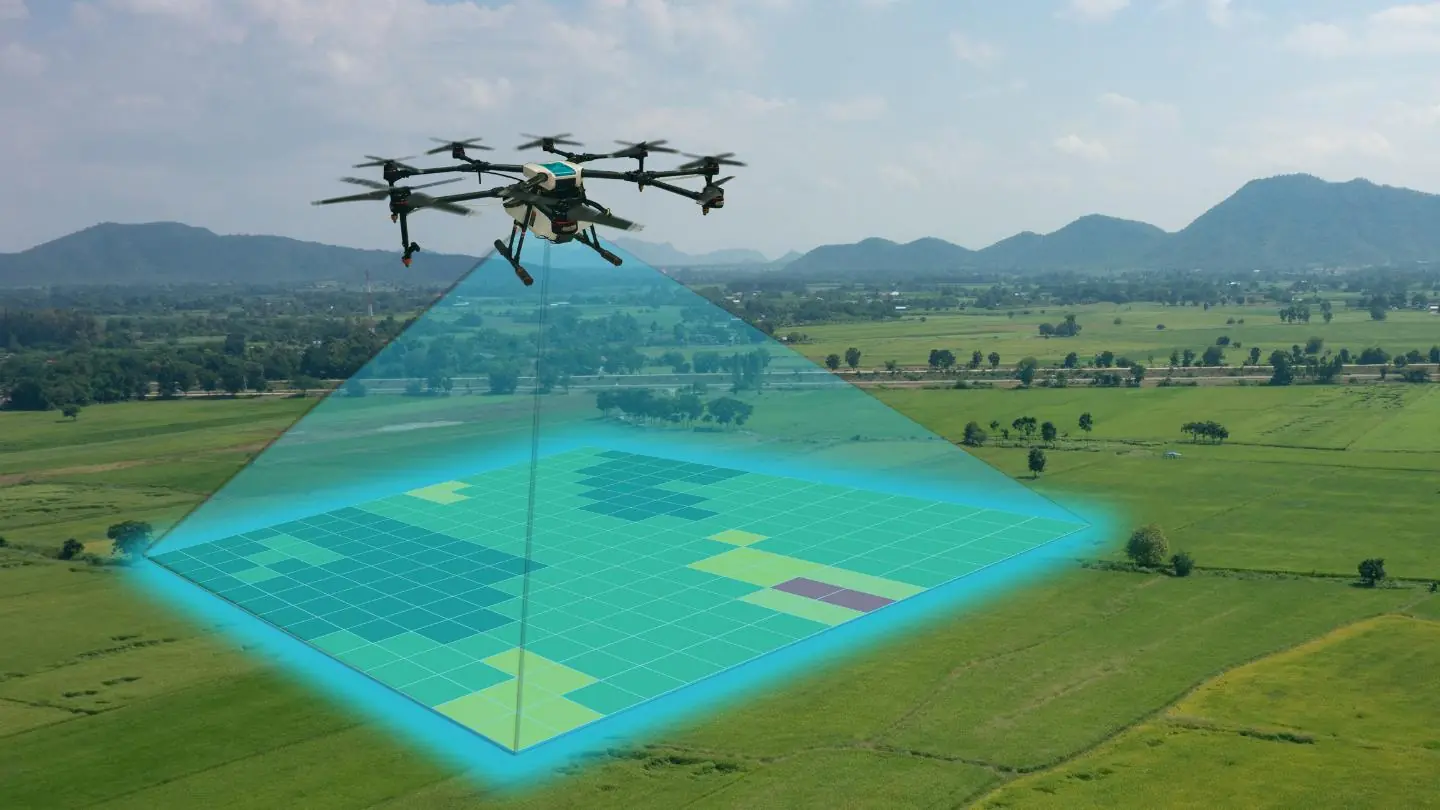
Crop Monitoring and Health Assessment
- Disease Detection: Drones or ground-based cameras collect high-resolution images. These are processed using computer vision models trained with annotated agricultural datasets. When symptoms like leaf spots or discoloration are detected, the system can flag areas needing attention.
- Precision Irrigation and Fertilization: Image-based systems can detect moisture levels in soil or leaf coloration that indicates nutrient status. This helps tailor water and fertilizer application precisely where it’s needed.
- Example: In a tomato field pilot, using labeled data to train a computer vision system that identifies early-stage fungal infections. The result: pesticide use dropped significantly due to targeted treatment.
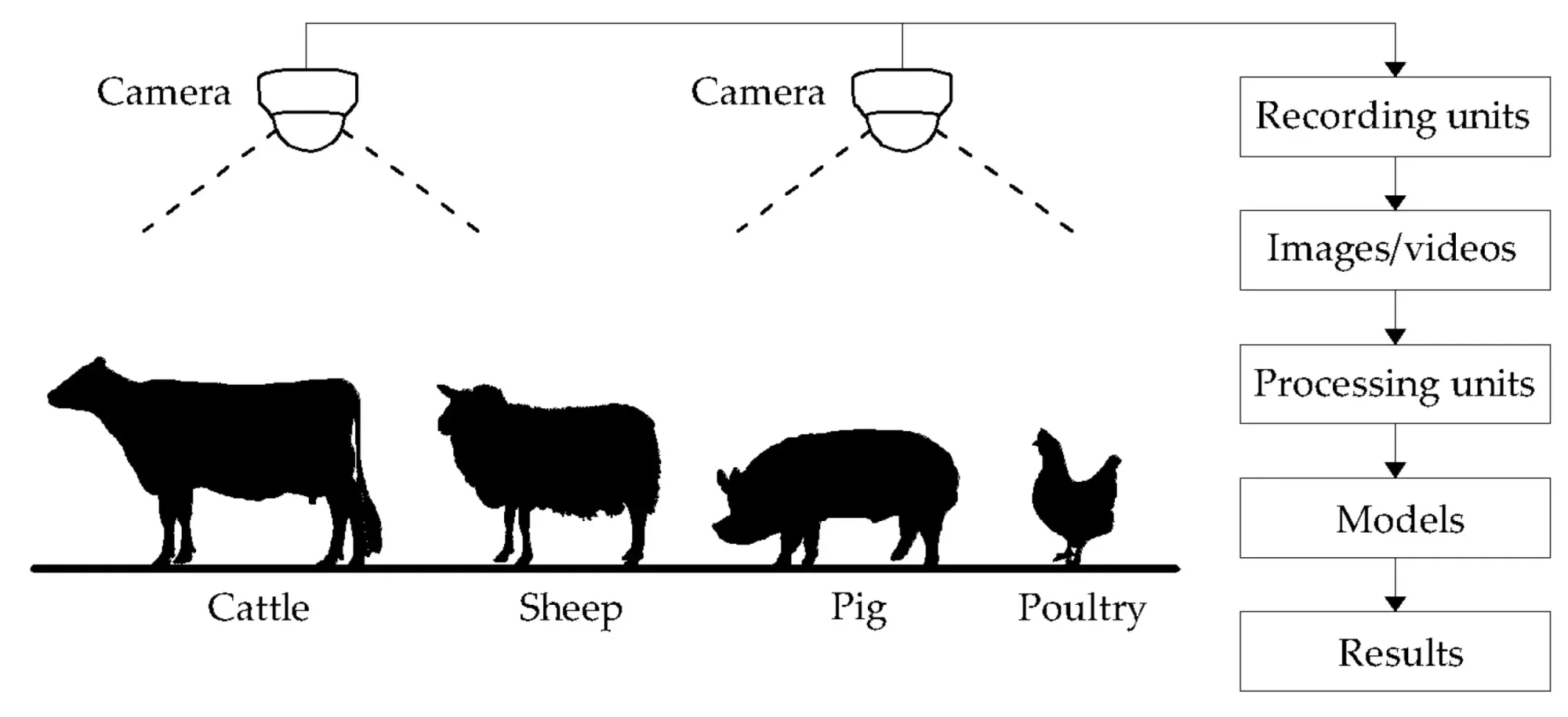
Livestock Management
- Health Tracking: Cameras track posture, gait, and activity levels. Annotated image data allows models to detect potential issues early.
- Behavior Analysis: Computer vision tools monitor feeding and movement patterns. Any deviations are flagged using pre-labeled data and classification models.
- Example: An AI-powered monitoring system, powered by IoT devices, observes dairy cow behavior and alerts farmers to signs of lameness or reduced feeding.
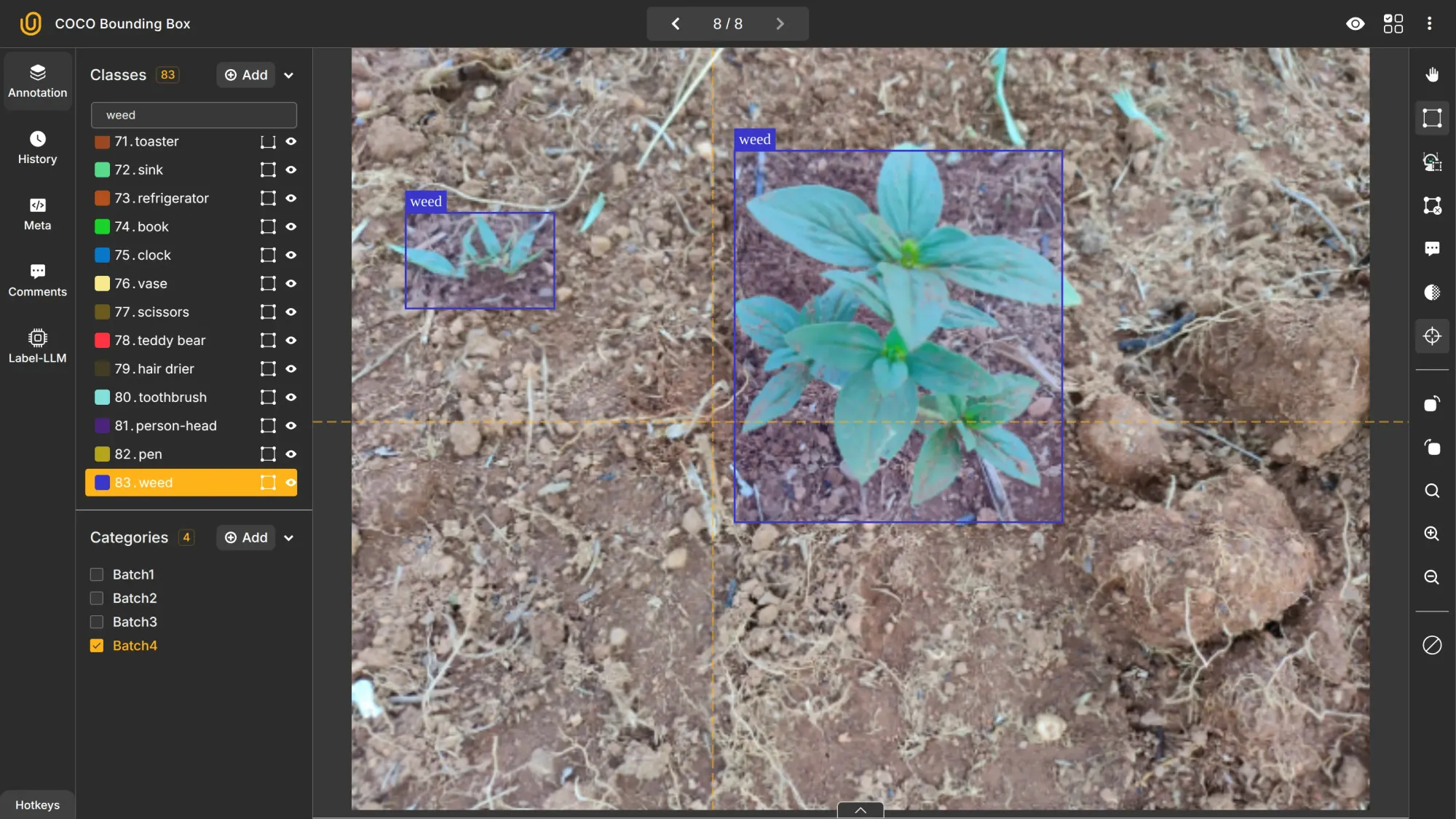
Precision Weed Control
- Automated Weed Identification: Vision systems trained with large, varied data can distinguish crops from weeds and either apply herbicide precisely or use mechanical removal.
- Scalable Field Solutions: These systems, paired with necessary hardware and infrastructure, can scale across tractors, drones, or autonomous units—ideal for operations of any size.
Benefits
-
Efficiency and Cost Reduction: Automated systems trained on high-quality annotated datasets reduce labor needs, eliminate guesswork, and make optimal use of resources like water and pesticides.
-
Enhanced Crop Quality: Thanks to accurate data labeling and timely interventions, farmers can expect healthier, more consistent produce—leading to higher market value.
-
Environmental Sustainability: With precise targeting made possible by AI-driven insights, farms can significantly cut back on chemical usage and water waste.
-
Data-Driven Insights: Insights from well-managed ML models support forecasting, market planning, and risk mitigation strategies for long-term success.
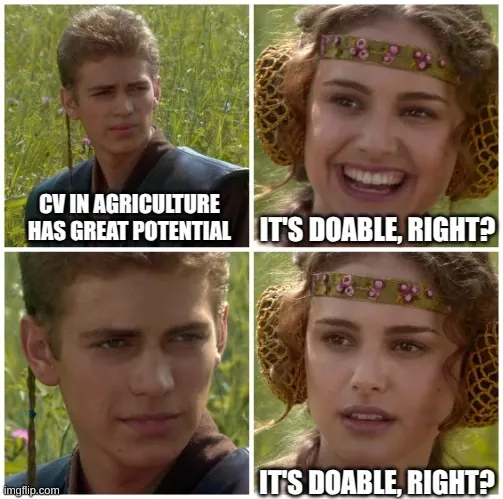
Challenges
-
Technical Barriers
- Data Quality: Training reliable models depends on high-quality image annotation and data labeling. Capturing and managing such diverse visual datasets can be labor-intensive.
- Infrastructure: Without stable internet access, syncing large annotated datasets or running real-time models becomes a challenge—especially in rural areas.
-
Financial Constraints
- Initial Investment: Acquiring cameras, drones, and computing infrastructure, along with premium data labeling services, may be cost-prohibitive for small farms.
- Ongoing Maintenance: Systems require updates, re-labeling, and fine-tuning using new datasets and version control processes, all of which demand time and expertise.
-
Regulatory and Ethical Considerations
- Drone Regulations: Legal frameworks vary by region, and acquiring drone licenses can slow down adoption.
- Data Ownership: As farms generate large datasets—often processed by third-party data annotation services—ownership and privacy become crucial concerns.
-
Skills and Training
- Learning Curve: Many farmers are not yet familiar with image labeling tools or dataset management systems. Education and onboarding are essential.
- Talent Shortage: Bridging the gap between agricultural expertise and technical know-how in dataset version control and annotation requires new kinds of cross-functional teams.
Future Outlook
As 5G and rural internet access improve, real-time data processing will become practical even in remote farming areas. We’re likely to see greater use of autonomous systems—powered by AI datasets and auto-labeling tools—that plant, nurture, and harvest crops with little human input.
Expect stronger collaboration between governments, agritech companies, and research institutions to standardize and expand access to dataset management platforms and data labeling services. As tools become more user-friendly and affordable, adoption will grow across the entire agricultural spectrum.
Conclusion
Computer vision—supported by smart image annotation, data labeling, and dataset management—offers a practical path to solving agriculture’s most pressing challenges. From identifying pests early to reducing chemical usage and predicting crop yields, these technologies are transforming farming into a more efficient, data-driven, and sustainable practice.
With continued innovation and investment in auto-labeling tools, AI datasets, and user education, precision agriculture is moving from the exception to the standard—unlocking a future where farms are smarter, cleaner, and more productive.
Explore More
Check out these resources for more on practical computer vision:
- Computer Vision in Healthcare: Applications, Benefits, and Challenges
- Practical Computer Vision: Parking Lot Monitoring
- The Transformative Power of Computer Vision in Logistics
References
- Arslan Muhir et al. (Sep 2024). Computer vision in smart agriculture and precision farming: Techniques and applications. Artificial Intelligence in Agriculture: https://www.sciencedirect.com/science/article/pii/S2589721724000266
- Ramūnas Berkmanas (Aug 5, 2024). Computer Vision in Agriculture: TOP Use Cases. EasyFlow Tech: https://easyflow.tech/computer-vision-in-agriculture/