In our previous post, we shared strategies to speed up your image annotation workflows. Among these strategies, auto labeling tools stood out as one of the most effective ways to save time and improve efficiency.
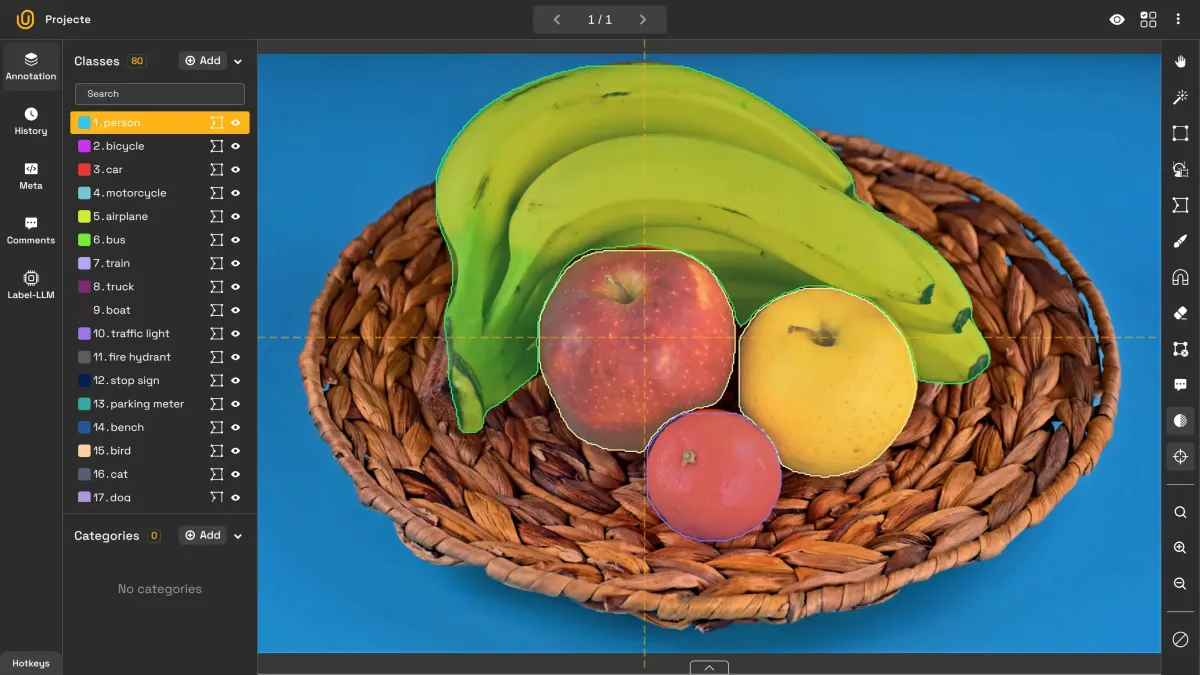
Accelerate Your Image Labeling Process | Unitlab Annotate
In this post, we’ll share 5 essential tips to help you get the most out of data auto-annotation and image labeling tools, making your data annotation process faster and more accurate.
1. Leverage Built-in AI-Assisted Labeling Tools
Most modern image annotation solutions offer built-in AI models that streamline tasks like object detection, semantic segmentation, and image classification. These tools are designed to automate common repetitive work, providing a cost-effective and efficient way to label your image datasets. You can usually explore what kind of AI-powered tools these data annotation platforms offer in their platforms.
AI-powered auto-annotation tools come in a great variety to cater to many different use cases and projects. Depending on your use case, you may want to use one type of image annotation, which determines your use of tool. For example, to differentiate each object in the image, we can use crop auto-annotation that automatically detects and classifies objects:
Crop Auto-annotation | Unitlab Annotate
Unitlab Annotate includes robust pre-trained, AI-powered auto-labeling tools that can handle most common data annotation scenarios accurately with minimal effort. Whether you’re managing large AI datasets or working with smaller ML datasets, these tools can significantly reduce manual labeling work.
2. Integrate Your Own AI Models
While built-in AI models provided by data annotation platforms are excellent for general use cases, they may not address specialized needs. If your image data annotation involves niche tasks, such as rare object detection or industry-specific terminology, integrating your own AI models can be exceptionally valuable. The integration of your custom AI models can bring benefits of model visualization, model evaluation, and many others that come with a data annotation platform, such as dataset management and collaborative development.
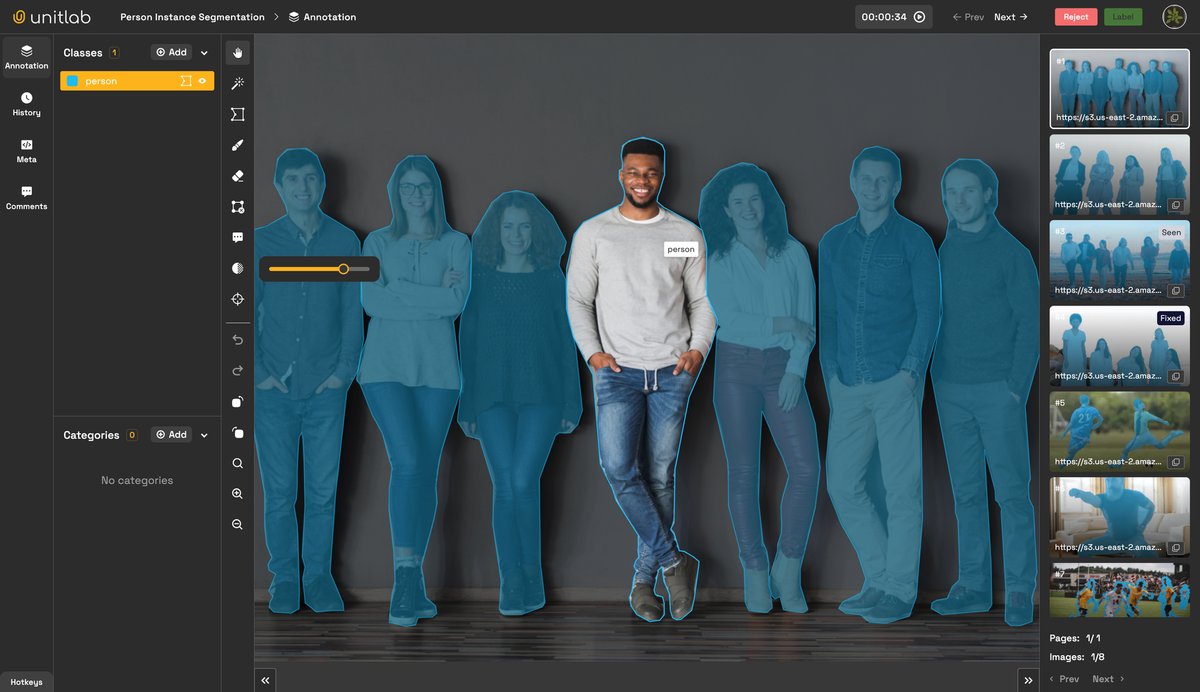
YOLOv8 Integration with Unitlab Annotate
Unitlab Annotate allows you to incorporate custom models tailored to your requirements. Train these AI models using high-quality labeled data, and then deploy them for tasks like image auto-labeling. Over time, as you refine the model’s outputs in Unitlab Annotate by reviewing them, you’ll create a feedback loop that enhances both accuracy and efficiency.
3. Utilize Batch Processing for Large Datasets
For teams working with large datasets, batch processing is an essential feature. It allows you to label entire groups of data—whether images or text—in a single operation, or batch, hence the name. This is particularly useful when dealing with large-scale image labeling solutions.
Image OCR Batch Auto-Annotation | Unitlab Annotate
To maximize the efficiency of this approach, organize your data into logical groups—such as images with similar lighting or text from related contexts. In other words, if you are interested in annotating vehicles, ensure that vehicles are the main component in your images. This ensures the image labeling tool applies consistent and accurate labels across the dataset, saving time and reducing the need for corrections.
4. Combine Auto-Labeling with Human Verification
While auto-labeling tools have advanced significantly in terms of accuracy, human verification still remains essential for maintaining quality. AI may struggle with edge cases or ambiguous data, so adding a human-in-the-loop step ensures that your annotations meet the highest standards without you losing the efficiency. A human data annotator reviewing the annotations of an AI model is both efficient and accurate than manual image annotation.
Unitlab Annotate includes the Reviewer
role who reviews and corrects annotations inside the image. By focusing human efforts on the quality control part, you can create a balance between automation and accuracy. This hybrid approach works especially well for complex image data annotation projects or large-scale data labeling services.
5. Set Up Clear Labeling Guidelines
Even with human reviewers in the loop, your labels may lack uniformity without clear, consistent rules, which reduces the overall quality of your dataset. Depending on the project and image annotation tasks, the organization or managers should set up clear labeling guidelines for handling edge cases and ambigious situations during image annotation. Check out our post to set up your guidelines:
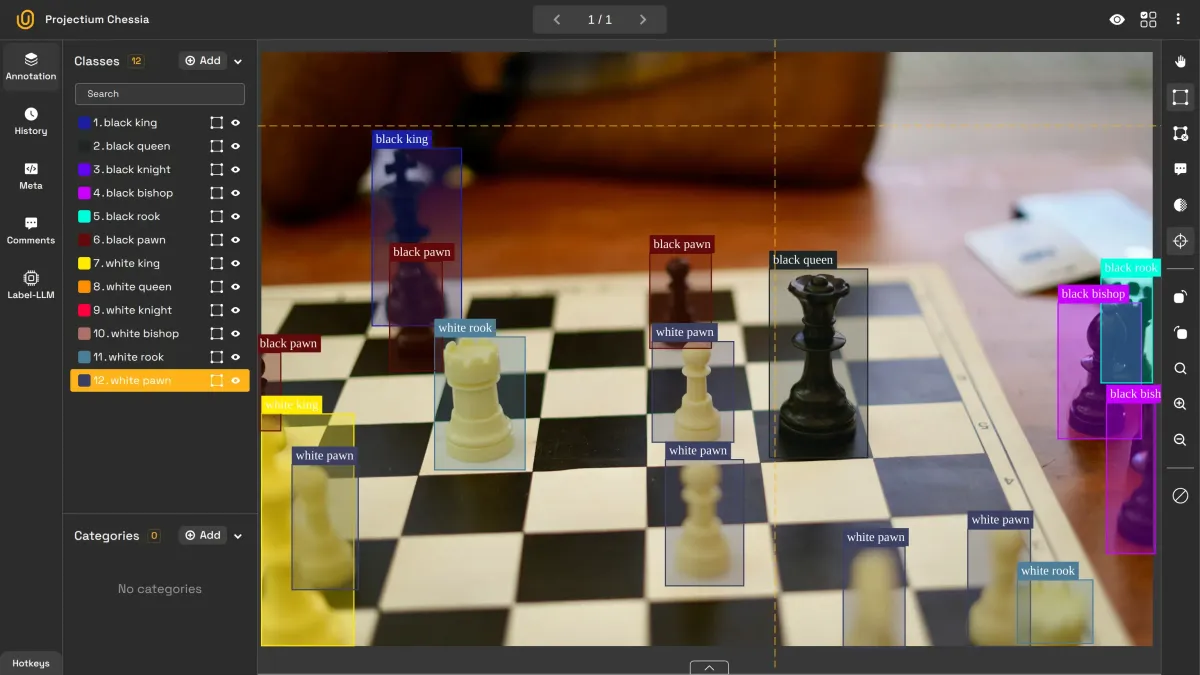
7 Tips for Accurate Image Labeling | Unitlab Annotate
Conclusion
Auto-labeling is a powerful tool that can dramatically accelerate data annotation and image labeling workflows, but success depends on using it strategically. By following these tips, you can optimize your process and ensure high-quality results.
Start by leveraging built-in auto-labeling tools for quick wins, integrate your own AI models for niche tasks, and use batch processing to handle large AI datasets efficiently. Pair automation with human verification for a hybrid approach that balances speed and accuracy, and always set up clear guidelines to ensure consistency.
Ready to transform your labeling process? Discover how Unitlab Annotate can support your projects with cutting-edge image labeling solutions, advanced dataset management, and flexible auto-labeling tools.