In our last post about Unitlab’s vision for this year, we highlighted our goal to unify “man and machine,” drawn from Zero to One by Peter Thiel. Our vision consists of these three steps:
- AI-powered automatic image labeling
- Human data annotator to correct any mistakes in auto-annotated images
- Human data reviewer to verify the final output, serving as a last quality check
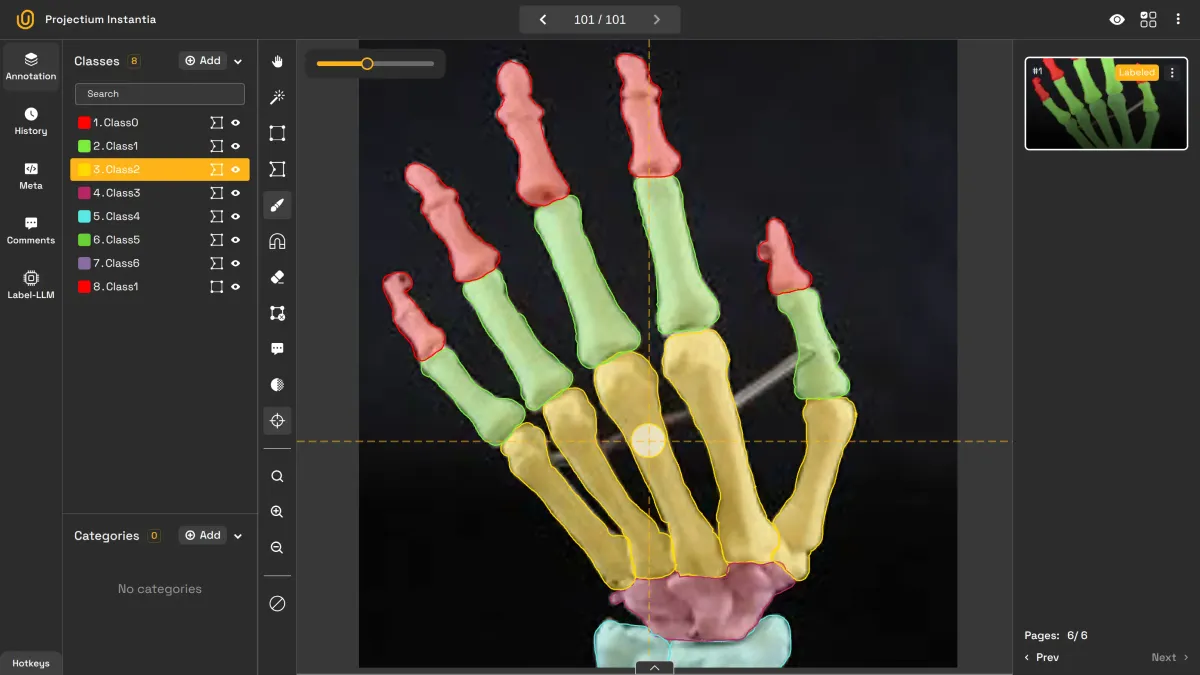
How We Transform Data Annotation | Unitlab Annotate
We explained why we believe this hybrid method is superior to extremes (only human or only machine-powered image annotation) in terms of speed, consistency, quality, and resource efficiency.
In this post, we’ll see how this approach works in practice within Unitlab Annotate, an innovative data annotation platform aiming to transform how images are labeled.
Introduction
As we mentioned before, merging AI-powered auto labeling tools with human image annotators produces results neither side can achieve alone. Humans excel at context and rational thinking; machines excel at speed and deterministic tasks. Human annotators are more expensive to train and maintain, while auto-annotation models are cheaper to set up and run. However, each covers the weaknesses of the other—truly, they’re complementary.
Our hybrid process at Unitlab Annotate aims to balance these strengths to deliver faster, more affordable, and higher-quality ML datasets for your AI/ML needs. Unitlab Annotate provides AI models and the option to integrate your custom AI models. Your annotators can easily join our platform, or you can hire an expert team of labelers from Unitlab. Finally, human reviewers validate the completed annotations for quality.
Let’s walk through how it all works. You can sign up for free and follow along in our tutorial.
Our 3-step Process for Data Annotation
First, let’s create a demo project—Hybrid Approach Demo—to illustrate our process. This project is available within the public AI datasets of Unitlab Annotate, where you can clone and explore it. We’ll use Image Skeleton, a specialized type of image annotation for outlining the blueprint of objects. We’ll also choose an AI model so you can see exactly how this approach comes together. Alternatively, if you have a custom AI model, you can integrate it into Unitlab as well.
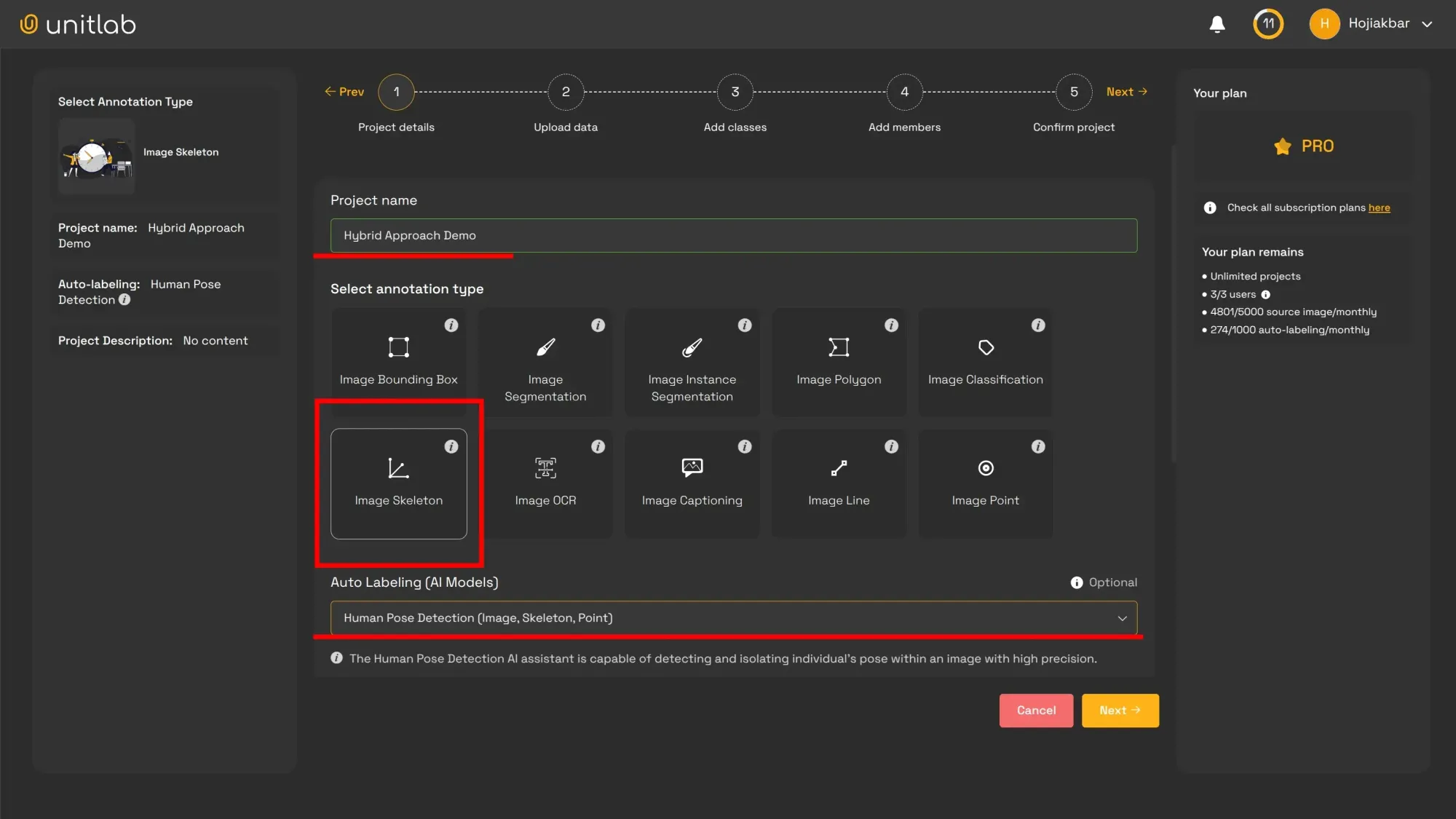
We’ll then upload the project and assign Annotator and Reviewer roles to team members. You can learn more about that in this post.
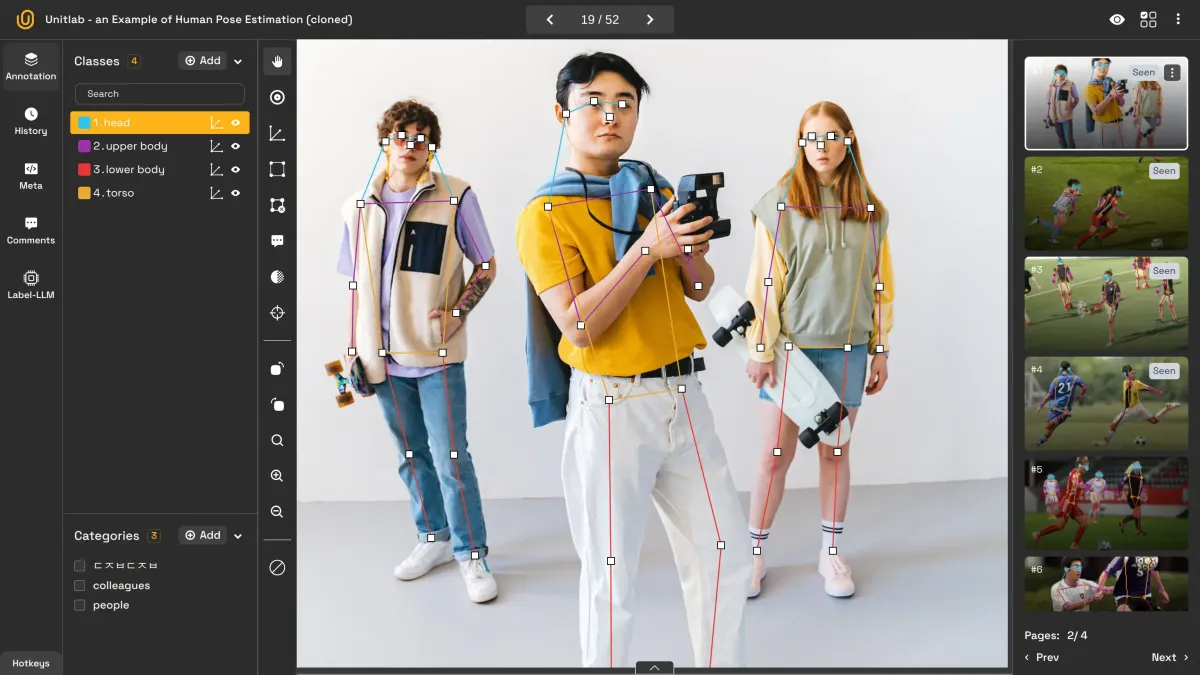
Project Creation at Unitlab | Unitlab Annotate
1. AI Auto-annotation
The first step in our hybrid approach is AI-powered auto-annotation. This swiftly and consistently handles image labeling, providing a baseline dataset for human annotators to refine. It can accelerate the initial image data annotation phase by a factor of 10 or even 100.
In short, auto labeling tools in our hybrid process:
- Annotate large image sets efficiently and uniformly
- Handle repetitive, mundane tasks
- Provide a reliable initial base for human refinement
In our Hybrid Approach Demo, we have 60 images of soccer/football players. With one command, we can run batch data auto-annotation in Unitlab Annotate platform:
Batch Human Pose Detection | Unitlab Annotate
2. Human Annotator Check
As fast and efficient as AI can be, it’s not infallible. AI is a set of deterministic algorithms that may fail in unexpected contexts or in areas requiring nuanced domain expertise (e.g., medical imaging). That’s why, after the automated tools finish labeling in a single batch, a human annotator steps in to refine and correct the results, ensuring the data labeling truly makes sense.
Human Labeler Fixing Mistakes | Unitlab Annotate
This step allows a trained data labeler to handle context and subtleties that machines miss.
- Refine and correct AI-generated annotations
- Adjust labels for context-specific nuances
- Bring human reasoning to ambiguous scenarios or novel cases
By letting machines handle the bulk of image labeling first, then having humans review, we get faster results with consistent quality—outperforming the slower, more error-prone approach of relying solely on humans.
3. Human Reviewer Check
The final step in our hybrid, man-machine approach is the human reviewer check. A reviewer is usually more experienced than the average labeler or AI model. This person reviews the data annotation work to confirm it follows guidelines and meets quality standards. They have the final say: accept or reject annotations, then comment on how to correct any issues.
Reviewer in Process | Unitlab Annotate
For this to work well, we need clear labeling guidelines. Unclear or shifting guidelines can frustrate annotators and hinder the process. As you can imagine, it’s demoralizing to have your work evaluated in a way that feels random or inconsistent.
In this final step, reviewers:
- Accept or reject completed annotations
- Cross-check for errors or inconsistencies
- Ensure final datasets meet predefined quality benchmarks
Reviewers serve as quality assurance managers in our data labeling service pipeline. They are responsible for providing clarity and maintaining a consistent, high-quality image annotation solution for AI/ML model development.
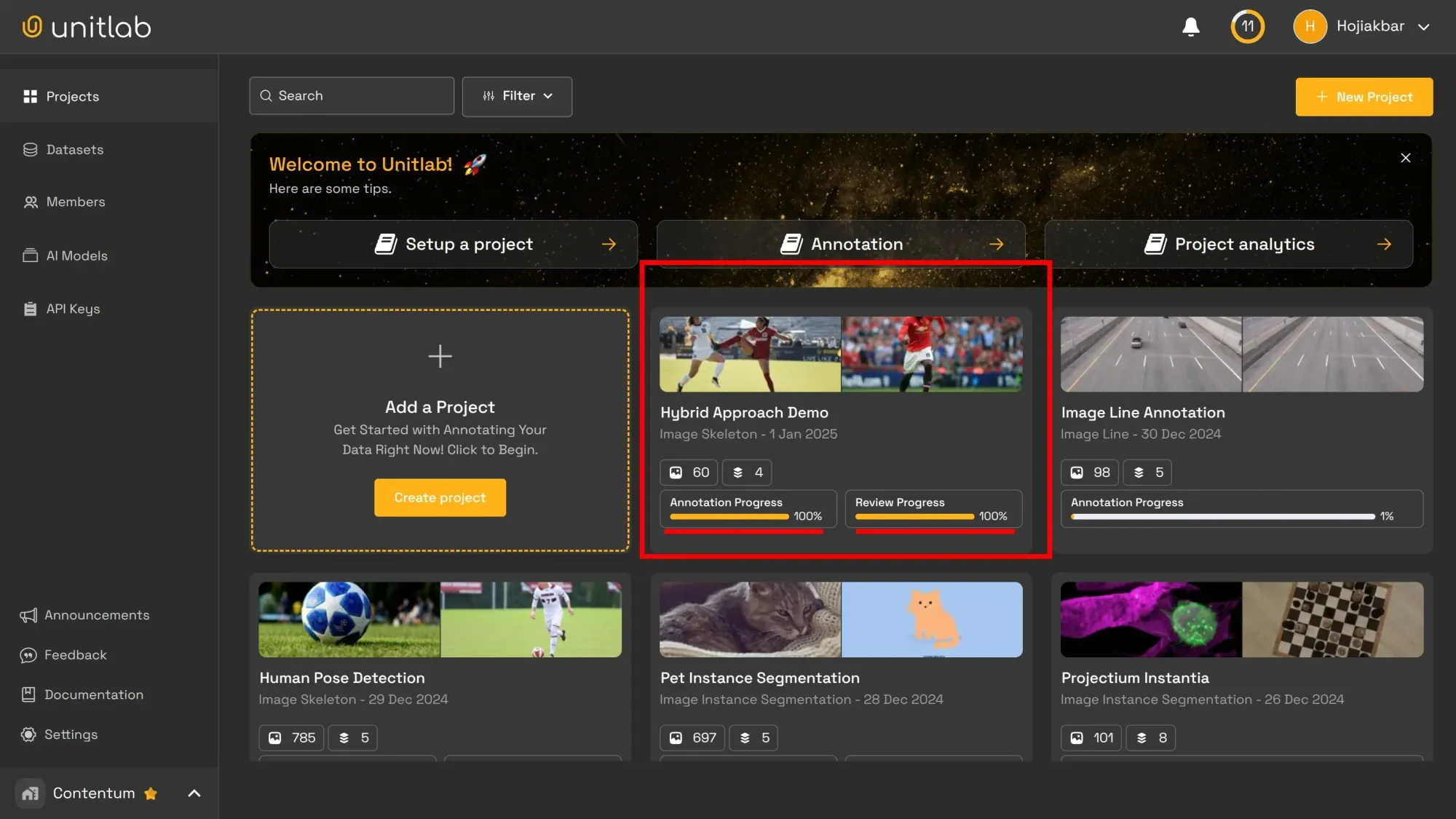
Releasing the Dataset
Once our demo project is done, we can release an initial version of it for the subsequent phases of AI/ML model development. This is also where dataset management and dataset version control come into play—ensuring you can track changes and maintain different versions of your AI datasets efficiently.
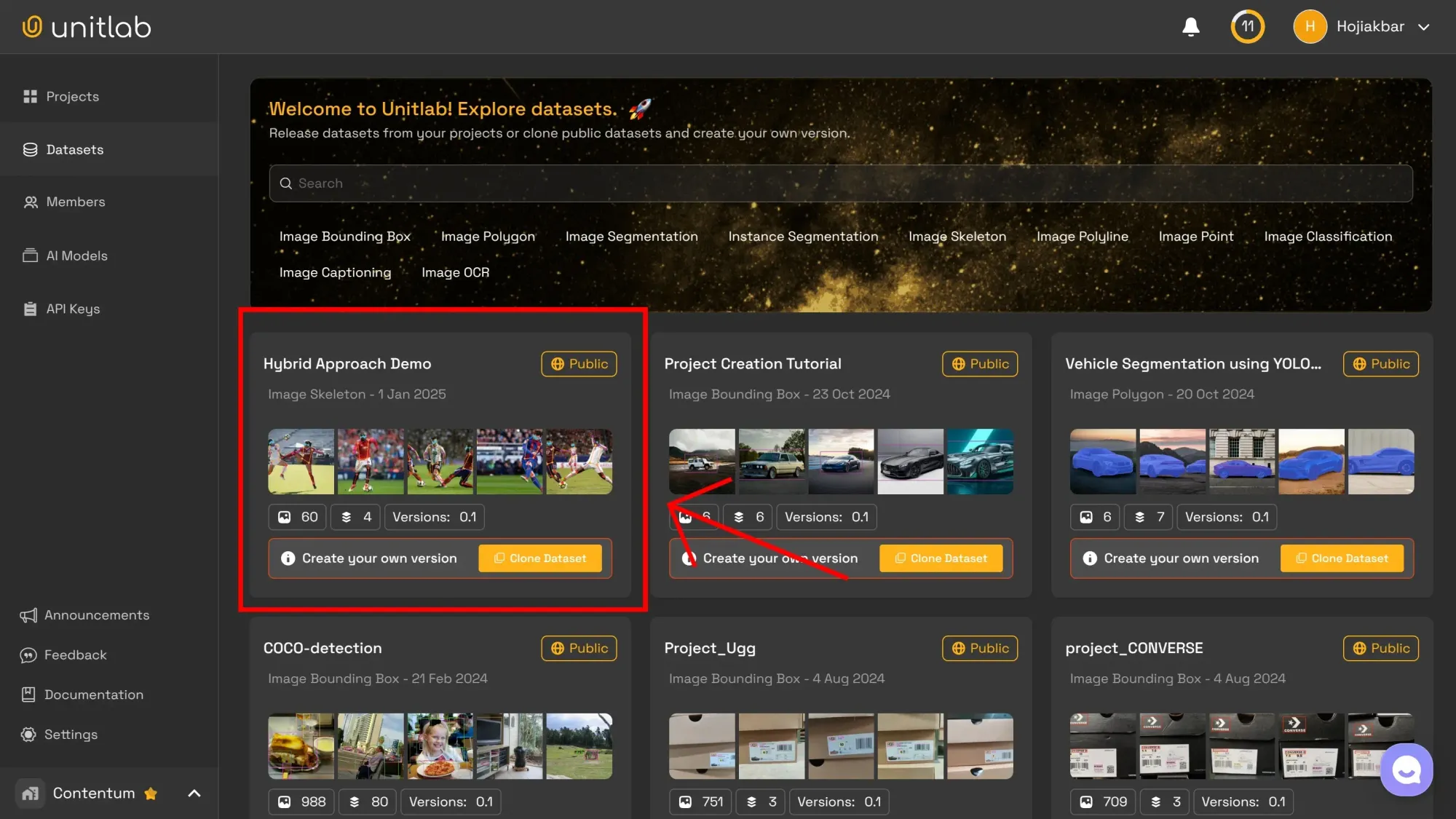
You can learn more about AI dataset management in our documentation and this blog post.
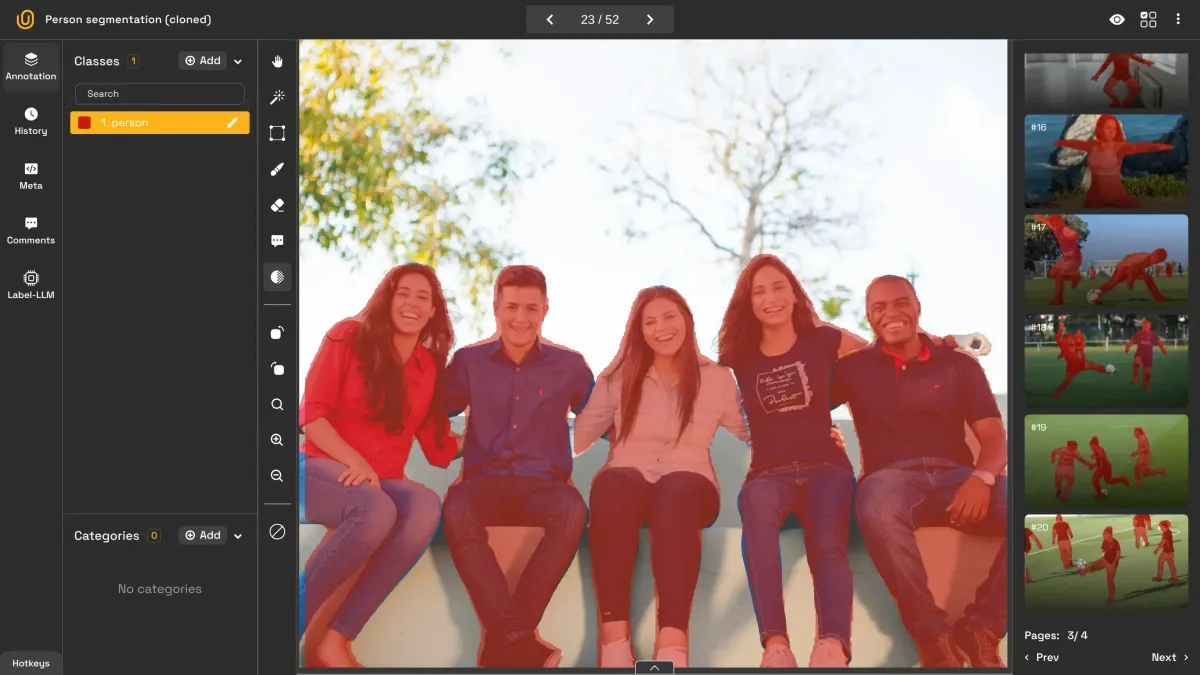
Dataset Management at Unitlab | Unitlab Annotate
Conclusion
We’ve demonstrated our balanced man-machine approach and explained why we believe it’s superior to relying solely on humans or solely on machines for image labeling service. At Unitlab Annotate, we walk the talk, offering a data annotation solution that provides a seamless experience for your team. Still not convinced? Try our platform for yourself and see the difference it makes in your image data annotation workflow.